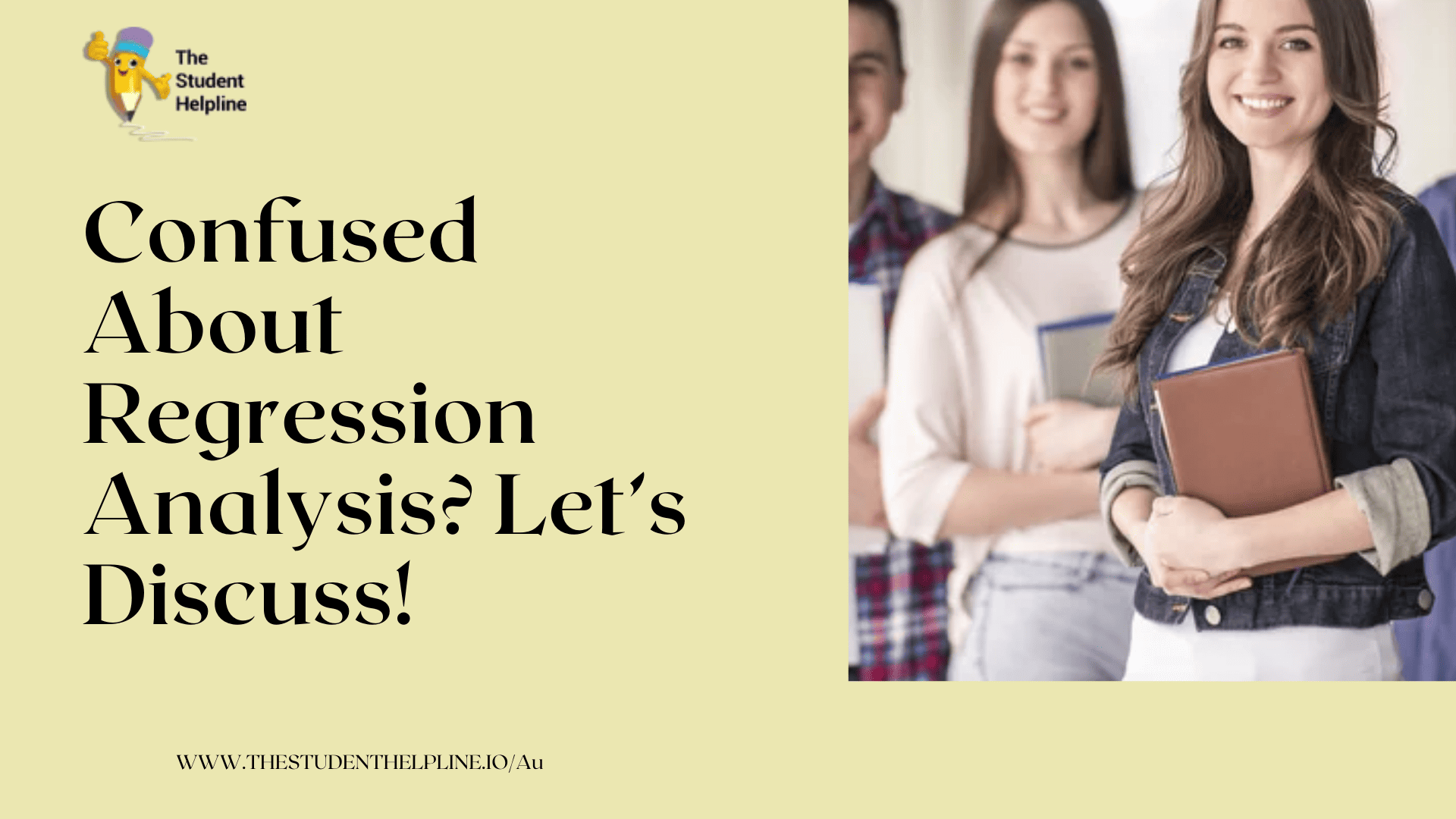
Regression analysis is a fundamental statistical technique used to model the relationship between a dependent variable and one or more independent variables. It is widely used in various fields, including economics, finance, social sciences, and engineering, to make predictions and understand the relationships between variables. If you are confused about regression analysis, you are not alone. Many students and professionals seek statistics assignment help to better understand and apply this powerful tool.
Understanding Regression Analysis
At its core, regression analysis aims to find the best-fitting line or curve that describes the relationship between variables. The most common type of regression analysis is linear regression, which assumes a linear relationship between the dependent variable (often denoted as ) and the independent variable(s) (denoted as ). The equation for a simple linear regression model is:
Here, is the intercept, is the slope, and is the error term, which accounts for the variability in that cannot be explained by .
Types of Regression Analysis
-
Simple Linear Regression: This involves one independent variable and one variable dependent. It is used to understand the relationship between the two variables and to make predictions based on that relationship.
-
Multiple Linear Regression: This extends simple linear regression by including more than one independent variable. It helps in understanding the combined effect of multiple factors on the dependent variable.
-
Polynomial Regression: This type of regression is used when the relationship between the dependent and independent variables is not linear but can be modeled by a polynomial function.
-
Logistic Regression: Used when the dependent variable is binary (e.g., yes/no, success/failure). It models the probability of the occurrence of an event by fitting data to a logistic curve.
Key Concepts in Regression Analysis
-
R-squared (RΒ²): This statistic indicates the proportion of the variance in the dependent variable that is predictable from the independent variable(s). An R-squared value close to 1 indicates a good fit of the model to the data.
-
P-values: These are used to test the significance of the regression coefficients. A low p-value (typically β€ 0.05) indicates that the coefficient is statistically significant, meaning it is unlikely to have occurred by chance.
-
Confidence Intervals: These provide a range of values for an unknown parameter, calculated from the data. They give an estimate of the precision of the regression coefficients.
-
Residuals: These are the differences between the observed values of the dependent variable and the values predicted by the regression model. Analyzing residuals can help identify patterns or outliers that might indicate problems with the model.
Common Challenges in Regression Analysis
-
Multicollinearity: This occurs when independent variables in a multiple regression model are highly correlated with each other. It can make it difficult to determine the individual effect of each variable on the dependent variable.
-
Heteroscedasticity: This refers to the condition where the variance of the error terms is not constant across all levels of the independent variables. This can lead to inefficient and biased estimates of the regression coefficients.
-
Overfitting: This happens when a model is too complex and fits the training data too closely, capturing noise rather than the underlying relationship. It can result in poor predictive performance on new data.
Seeking Statistics Assignment Help
If you are struggling with regression analysis, seeking statistics assignment help can be incredibly beneficial. Professional tutors and online resources can provide guidance on understanding the concepts, interpreting results, and solving problems. They can also help you with software tools like R, Python, SPSS, or Excel, which are commonly used for regression analysis.
Practical Tips for Regression Analysis
-
Data Exploration: Before running a regression analysis, explore your data using descriptive statistics and visualizations. This can help you understand the distribution of your variables and identify any potential issues.
-
Model Selection: Choose the appropriate type of regression model based on the nature of your data and the research question. Consider the assumptions of each model and whether they are met.
-
Validation: Validate your model by checking the assumptions and using techniques like cross-validation to ensure it performs well on unseen data.
-
Interpretation: Carefully interpret the results of your regression analysis. Pay attention to the coefficients, p-values, and R-squared values to understand the significance and strength of the relationships.
Conclusion
Regression analysis is a powerful statistical tool that can provide valuable insights into the relationships between variables. While it can be complex and challenging, understanding the key concepts and seeking statistics assignment help when needed can make the process more manageable. By mastering regression analysis, you can enhance your ability to make informed decisions and predictions based on data.
Leave a Reply